Machine Learning (ML) has emerged as a game-changing technology within the field of Artificial Intelligence (AI). In this brief summary, we will explore the essence of machine learning, its fundamental components, notable applications, and the promising implications it holds for the future.
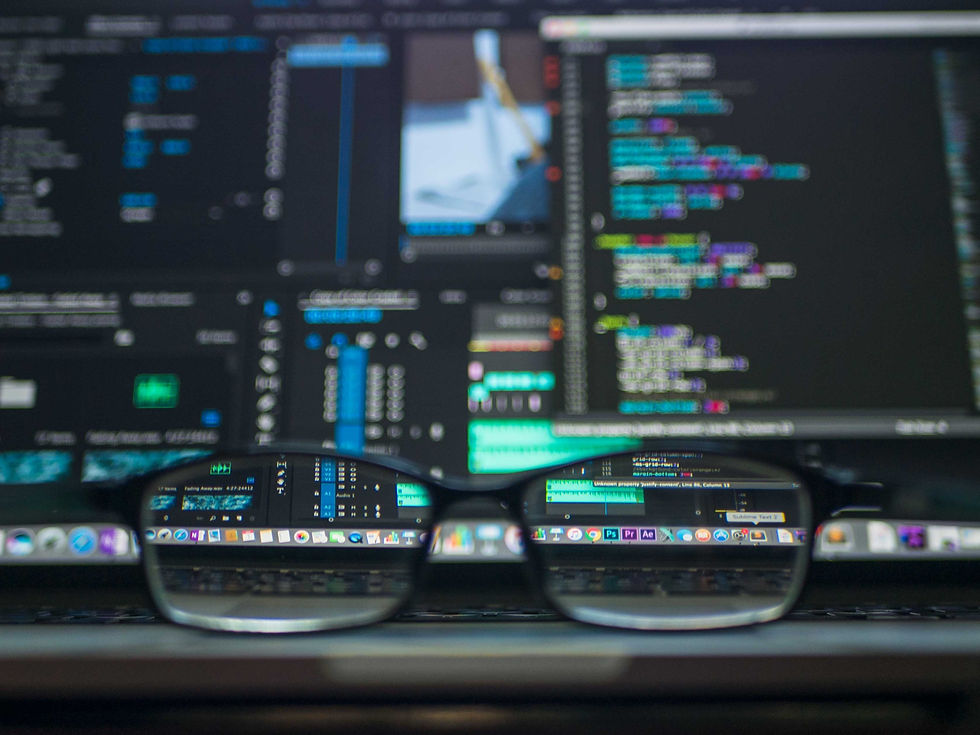
Understanding Machine Learning: Machine Learning refers to the capability of computer systems to learn from data and improve their performance without explicit programming. By analyzing vast amounts of data, ML algorithms can identify patterns, make predictions, and derive meaningful insights.
Key Components of Machine Learning: a) Training Data: ML algorithms require labeled training data to learn from. This data serves as examples for the algorithms to identify patterns and make accurate predictions.
a) Understanding Machine Learning: Machine Learning refers to the capability of computer systems to learn from data and improve their performance without explicit programming. By analyzing vast amounts of data, ML algorithms can identify patterns, make predictions, and derive meaningful insights.
b) Key Components of Machine Learning: a) Training Data: ML algorithms require labeled training data to learn from. This data serves as examples for the algorithms to identify patterns and make accurate predictions.
Applications of Machine Learning: a) Image and Speech Recognition: ML has significantly advanced image and speech recognition systems, enabling applications such as facial recognition, object detection, and voice-to-text conversion.
b) Natural Language Processing: ML algorithms power chatbots, language translation, sentiment analysis, and text summarization, enabling computers to understand and generate human language effectively.
c) Recommender Systems: ML analyzes user preferences and behavior to provide personalized recommendations in domains like e-commerce, entertainment, and content streaming platforms.
d) Healthcare and Medicine: ML plays a vital role in disease diagnosis, outcome prediction, drug discovery, and personalized medicine, assisting healthcare professionals in making data-driven decisions.
e) Autonomous Vehicles: ML is crucial for self-driving cars, enabling them to analyze sensor data, perceive the environment, and make real-time decisions for safe navigation.
f) Fraud Detection and Cybersecurity: ML algorithms aid in detecting fraudulent activities, identifying patterns in network traffic for intrusion detection, and strengthening cybersecurity measures.
Future Implications of Machine Learning: a) Advancements in Deep Learning: Continued research in deep learning techniques, such as Convolutional Neural Networks (CNNs) and Recurrent Neural Networks (RNNs), will unlock new possibilities for handling complex data and achieving state-of-the-art results.
b) Enhanced Decision-Making: ML-driven insights and predictive analytics will enable organizations to make data-driven decisions, optimize operations, and improve efficiency across various industries.
c) Personalized Experiences: ML-powered personalization will continue to enhance user experiences through tailored recommendations, customized services, and targeted advertisements.
d) Ethical Considerations: As ML becomes more pervasive, careful attention to ethical considerations, such as data privacy, bias mitigation, and transparency, will be crucial to ensure fairness and accountability.
Conclusion
Machine Learning has become a catalyst for transformation across industries, offering remarkable potential for the future. Its applications span from healthcare and finance to transportation and cybersecurity. As advancements in algorithms, computing power, and data availability continue, machine learning will empower innovation, drive progress, and shape a future where data-driven decision-making is the norm. Responsible development and ethical implementation will be essential to maximize the benefits of machine learning while addressing potential challenges and ensuring a positive impact on society.
15% DISCOUNT